Instructor condujo el entrenamiento
Machine Learning with Python
La formación dirigida por un instructor (ILT) es una forma tradicional de educación que implica que un instructor cualificado dirija un aula o una sesión virtual para impartir formación a los alumnos.
Plazas limitadas disponibles, inscríbete antes de la fecha June 09, 2025.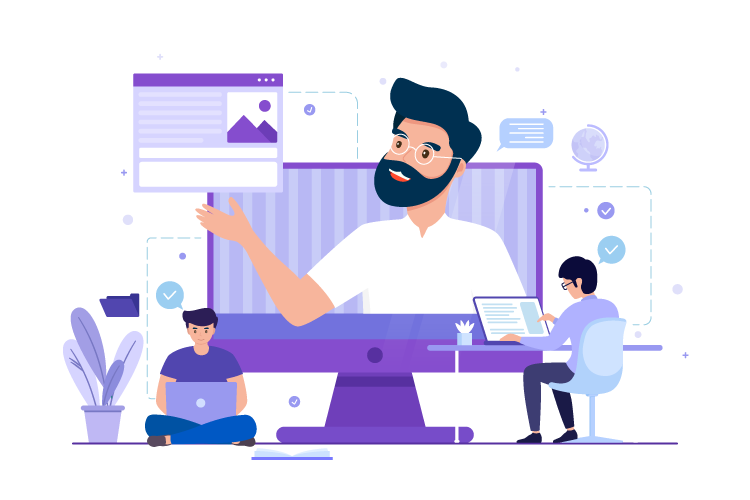
¿Por qué debería comprar un curso dirigido por un instructor?
Invertir en un curso dirigido por un instructor ofrece varias ventajas que pueden mejorar enormemente su experiencia de aprendizaje. Uno de los beneficios clave es la oportunidad de recibir orientación experta de profesionales experimentados que poseen un amplio conocimiento y experiencia en el tema. Estos instructores pueden ofrecer información valiosa, abordar sus consultas y brindar orientación adaptada a sus necesidades específicas. Además, los cursos dirigidos por un instructor siguen un plan de estudios bien estructurado, lo que garantiza un viaje de aprendizaje integral que cubre todos los temas esenciales. Este enfoque estructurado le permite progresar de manera lógica y organizada, construyendo una base sólida de conocimientos. Además, los cursos dirigidos por un instructor a menudo brindan comentarios personalizados, lo que le permite recibir evaluaciones y orientación individualizadas para mejorar su comprensión y sus habilidades.
Certificado profesional.
Obtener una certificación de finalización es un beneficio importante que se obtiene con muchos cursos dirigidos por un instructor. Esta certificación sirve como reconocimiento formal de su finalización exitosa del curso y muestra su compromiso con el aprendizaje y el desarrollo profesional. Puede ser una valiosa adición a su currículum o cartera, destacando su experiencia y dedicación en un campo o conjunto de habilidades específico. La certificación demuestra a empleadores, clientes o colegas que ha adquirido los conocimientos y habilidades necesarios para realizar tareas de manera efectiva. Puede mejorar su credibilidad y abrir puertas a nuevas oportunidades o avances profesionales. Además, la certificación brinda una sensación de logro y satisfacción, validando el tiempo y el esfuerzo invertido en el curso. En última instancia, la certificación de finalización ofrece evidencia tangible de su compromiso con el aprendizaje continuo y el crecimiento profesional, lo que la convierte en un activo valioso en el competitivo mercado laboral actual.
¿Como funciona?
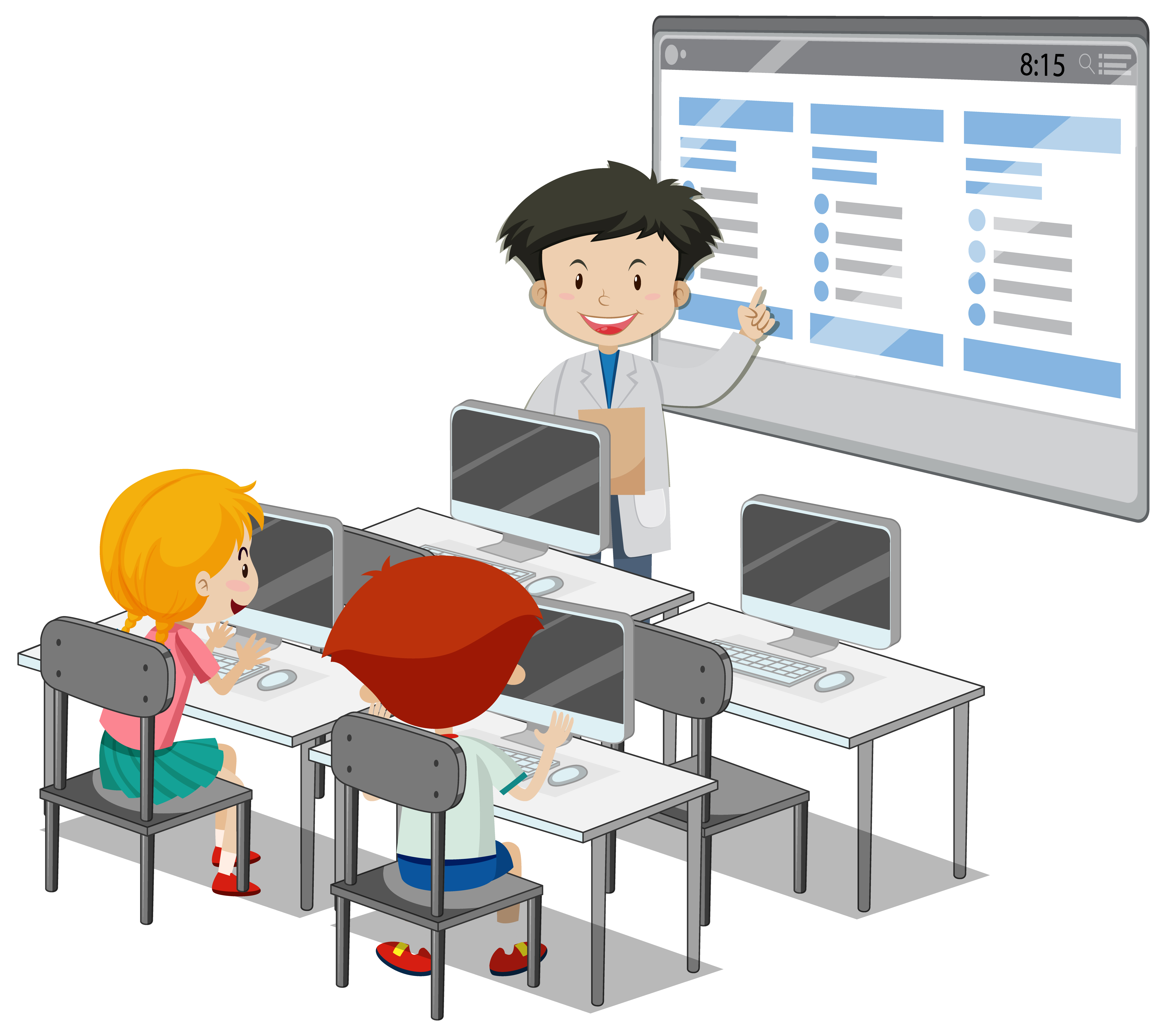
Reunión de Zoom con el estudiante dos veces por semana.
Como educador, he implementado un enfoque de aprendizaje estructurado mediante la realización de reuniones de Zoom con mis alumnos dos veces por semana. Esta plataforma interactiva se ha convertido en una herramienta invaluable para fomentar conexiones significativas y facilitar debates interesantes en un aula virtual.
Soporte de tutores de IA.
El apoyo de la tutoría desempeña un papel crucial a la hora de guiar a las personas hacia el crecimiento personal y profesional. Al ofrecer tutoría, proporciono un espacio seguro y de apoyo para que las personas exploren sus metas, desafíos y aspiraciones.
Tareas y calificaciones.
Las tareas y las calificaciones son componentes esenciales del proceso educativo, ya que permiten a los estudiantes demostrar su comprensión de conceptos y habilidades y, al mismo tiempo, brindan a los maestros un medio para evaluar su progreso. Las tareas están diseñadas para reforzar el aprendizaje, fomentar el pensamiento crítico y promover la resolución independiente de problemas.
Acerca de este curso
Learn Machine Learning with Python, a comprehensive training manual that teaches the fundamentals of coding with Python. Whether you want to improve your coding skills or you want an upgrade at your workplace, this is the ideal start. In this course, you’ll master the processes, patterns, and strategies of this user-friendly programming language. This Python ML course covers supervised learning paradigms, like classical algorithms, and regression techniques to evaluate performance metrics. Besides this, you’ll also learn feature engineering for converting raw data into meaningful features. Furthermore, you’ll also leverage the Python scikit-learn library along with other powerful tools. Practice on our Labs to solidify your understanding as you explore object-oriented programming, modules, error handling, and even file operations. By the end of this course, you'll be confidently writing Python ML scripts and resolving coding issues.
Habilidades que obtendrás
Plan de estudios
Let’s Discuss Learning
- Welcome
- Scope, Terminology, Prediction, and Data
- Putting the Machine in Machine Learning
- Examples of Learning Systems
- Evaluating Learning Systems
- A Process for Building Learning Systems
- Assumptions and Reality of Learning
- End-of-Lesson Material
Some Technical Background
- About Our Setup
- The Need for Mathematical Language
- Our Software for Tackling Machine Learning
- Probability
- Linear Combinations, Weighted Sums, and Dot Products
- A Geometric View: Points in Space
- Notation and the Plus-One Trick
- Getting Groovy, Breaking the Straight-Jacket, and Nonlinearity
- NumPy versus “All the Maths”
- Floating-Point Issues
- EOC
Predicting Categories: Getting Started with Classification
- Classification Tasks
- A Simple Classification Dataset
- Training and Testing: Don’t Teach to the Test
- Evaluation: Grading the Exam
- Simple Classifier #1: Nearest Neighbors, Long Distance Relationships, and Assumptions
- Simple Classifier #2: Naive Bayes, Probability, and Broken Promises
- Simplistic Evaluation of Classifiers
- EOC
Predicting Numerical Values: Getting Started with Regression
- A Simple Regression Dataset
- Nearest-Neighbors Regression and Summary Statistics
- Linear Regression and Errors
- Optimization: Picking the Best Answer
- Simple Evaluation and Comparison of Regressors
- EOC
Evaluating and Comparing Learners
- Evaluation and Why Less Is More
- Terminology for Learning Phases
- Major Tom, There’s Something Wrong: Overfitting and Underfitting
- From Errors to Costs
- (Re)Sampling: Making More from Less
- Break-It-Down: Deconstructing Error into Bias and Variance
- Graphical Evaluation and Comparison
- Comparing Learners with Cross-Validation
- EOC
Evaluating Classifiers
- Baseline Classifiers
- Beyond Accuracy: Metrics for Classification
- ROC Curves
- Another Take on Multiclass: One-versus-One
- Precision-Recall Curves
- Cumulative Response and Lift Curves
- More Sophisticated Evaluation of Classifiers: Take Two
- EOC
Evaluating Regressors
- Baseline Regressors
- Additional Measures for Regression
- Residual Plots
- A First Look at Standardization
- Evaluating Regressors in a More Sophisticated Way: Take Two
- EOC
More Classification Methods
- Revisiting Classification
- Decision Trees
- Support Vector Classifiers
- Logistic Regression
- Discriminant Analysis
- Assumptions, Biases, and Classifiers
- Comparison of Classifiers: Take Three
- EOC
More Regression Methods
- Linear Regression in the Penalty Box: Regularization
- Support Vector Regression
- Piecewise Constant Regression
- Regression Trees
- Comparison of Regressors: Take Three
- EOC
Manual Feature Engineering: Manipulating Data for Fun and Profit
- Feature Engineering Terminology and Motivation
- Feature Selection and Data Reduction: Taking out the Trash
- Feature Scaling
- Discretization
- Categorical Coding
- Relationships and Interactions
- Target Manipulations
- EOC
Tuning Hyperparameters and Pipelines
- Models, Parameters, Hyperparameters
- Tuning Hyperparameters
- Down the Recursive Rabbit Hole: Nested Cross-Validation
- Pipelines
- Pipelines and Tuning Together
- EOC
Combining Learners
- Ensembles
- Voting Ensembles
- Bagging and Random Forests
- Boosting
- Comparing the Tree-Ensemble Methods
- EOC
Models That Engineer Features for Us
- Feature Selection
- Feature Construction with Kernels
- Principal Components Analysis: An Unsupervised Technique
- EOC
Feature Engineering for Domains: Domain-Specific Learning
- Working with Text
- Clustering
- Working with Images
- EOC
Connections, Extensions, and Further Directions
- Optimization
- Linear Regression from Raw Materials
- Building Logistic Regression from Raw Materials
- SVM from Raw Materials
- Neural Networks
- Probabilistic Graphical Models
- EOC
Appendix A: mlwpy.py Listing
preguntas frecuentes
¿Listo para comenzar?